Le monde de l’internet a transformé la façon dont nous faisons nos achats. Cependant, l’essor du commerce en ligne a aussi ouvert la porte à une multitude d’escroqueries. Heureusement, des solutions comme le détecteur d’arnaques e-commerce peuvent vous aider à...
Welcome to
CIOTECHIE.COM
La technologie est une science ou une connaissance mise en pratique pour résoudre des problèmes ou inventer des outils utiles.

Mission & Vision
Informer de l’impact des Technologies sur notre Futur
La technologie est omniprésente dans notre vie quotidienne. De la télévision aux téléphones cellulaires, en passant par les ordinateurs portables et les tablettes, nous avons accès à une infinité d’informations et de divertissements. Les techologies ont considérablement changé la façon dont nous communiquons et interagissons avec le monde qui nous entoure.
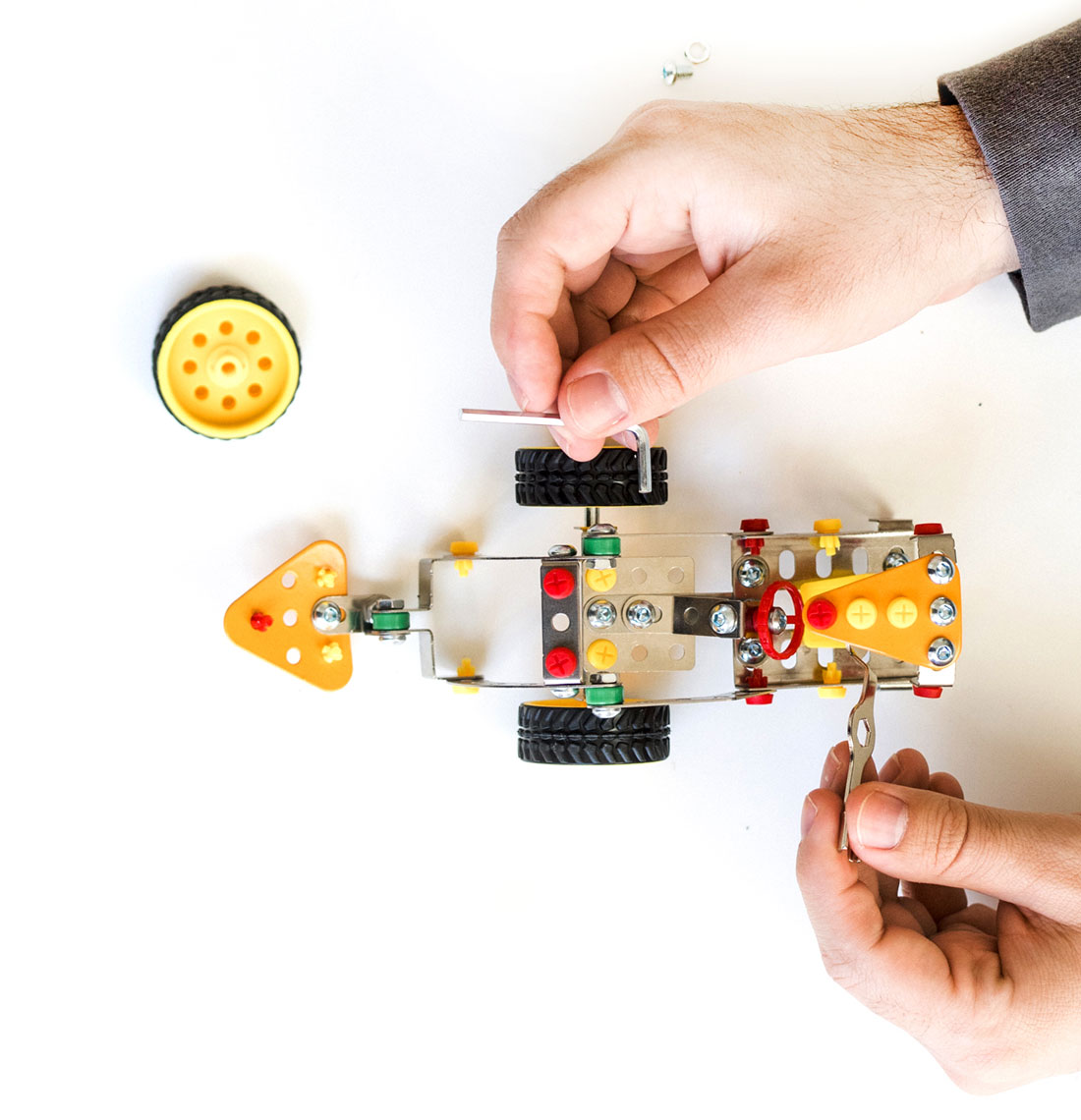

Education
Donner l’accès aux technologies à toutes les populations
Les gouvernements du monde entier ont pris conscience de l’importance d’offrir l’accès aux technologies à toutes les populations. Cela permet non seulement aux individus de bénéficier des avantages de la modernité, mais contribue également à réduire les inégalités sociales.
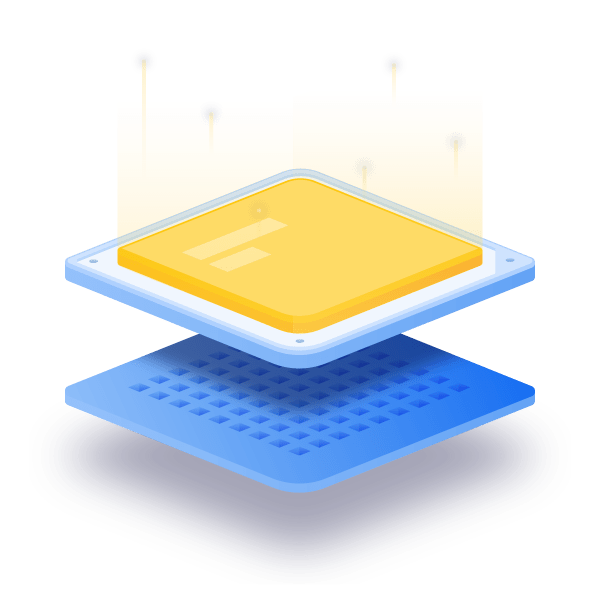
BLOG
Bénéficiez de notre expertise sur ces sujets
Technologie : Comment dynamiser la formation tech de vos équipes ?
Technologie : Comment dynamiser la formation tech de vos équipes ? Comment les entreprises peuvent-elles s'assurer que leurs équipes restent à la pointe de la connaissance ? La réponse pourrait bien résider dans la production de vidéos de formation spécifiquement...
Comment choisir une alarme maison pour plus de sécurité ?
Quelques années auparavant, seuls les propriétaires fortunés pouvaient se permettre d'installer une alarme maison sectoralarm. Aujourd'hui, le système de sécurité est devenu abordable et accessible à tous ceux qui veulent protéger leur maison. Un système d'alarme peut...
Les différents systèmes d’alarmes pour votre maison
Vous êtes à la recherche d’un système d’alarme sûr et fiable pour votre maison ? Il existe plusieurs options sur le marché, chacune avec ses avantages et ses inconvénients. Vous aurez le choix entre une alarme maison sectoralarm ou d'autres alarmes. Nous allons...
Sécurité d’une maison connectée : profitez de l’intelligence artificielle pour être en sécurité
Lorsqu'on parle de technologie et de sécurité dans le même contexte, on pense tout de suite aux systèmes antivirus, à des logiciels malveillants ou encore aux attaques extérieures. Mais la technologie s'est beaucoup développée ces dernières années, et les technologies...
Pourquoi la batterie de votre ordinateur portable gonfle ?
Les batteries des ordinateurs portables sont soumises à une usure normale et s'usent à mesure que vous en faites usage. Elles peuvent également s'abîmer ou surchauffer et parfois même se déformer, ce qui est le cas lorsque la batterie commence à gonfler. Dans cet...
Les avantages d’un moniteur amovible pour ordinateur portable
Avec le développement des technologies, les ordinateurs portables sont devenus une partie intégrale de nos vies. Ils offrent une polyvalence exceptionnelle et peuvent être utilisés à la maison et au travail. Mais pour certains usagers, un écran supplémentaire est...
Quelles sont les marques d’ordinateurs portables à éviter ?
Si vous êtes à la recherche d’un ordinateur portable, il peut s’avérer compliqué de choisir un modèle. Plusieurs critères entrent en compte : marque, praticité et budget… Alors que certaines marques offrent une très bonne qualité pour un prix abordable, d'autres...
L’Exercice d’Evaluation Pratique du Télépilote de Drone
De plus en plus de personnes sont attirées par l'utilisation des drones et des télépilotes. L'utilisation à des fins professionnelles est en forte croissance, mais elle requiert une formation spécifique et une certification pour obtenir le statut de pilote de drone...
Choisir un moteur de volet roulant Somfy : nos conseils
Les volets roulants sont une solution très prisée pour améliorer l'isolation thermique et acoustique d'une habitation. Cependant, choisir le bon moteur est une étape capitale pour bénéficier de tous les avantages de ce dispositif. Découvrez à travers cet article nos...
R & D
Recherche & Développement
Artificial Intelligence
Pour beaucoup, l’intelligence artificielle est quelque chose de mystérieux et de fascinant. Cependant, l’IA est également un sujet controversé, car il y a beaucoup de craintes concernant son impact sur l’emploi et la société dans son ensemble. Au fur et à mesure que l’IA se développe, il est important de prendre le temps de comprendre ce qu’elle peut apporter aux civilisations.
Machine Learning
Le machine learning est principalement utilisé dans les domaines de la reconnaissance faciale, de l’identification d’objets, de la reconnaissance de l’écriture manuscrite et de la reconnaissance vocale. Les applications potentielles du machine learning sont vastes et continuent d’être explorées.
Emerging Technologies
Parmi les technologies émergentes, on peut citer la réalité virtuelle, l’intelligence artificielle, les drones, les véhicules connectés, etc. Ces technologies ont le potentiel de changer radicalement notre façon de vivre et de travailler. Elles offrent de nouvelles perspectives aux entreprises et aux consommateurs.
